Revolutionizing Email Management: The Role of Spam Filtering Machine Learning
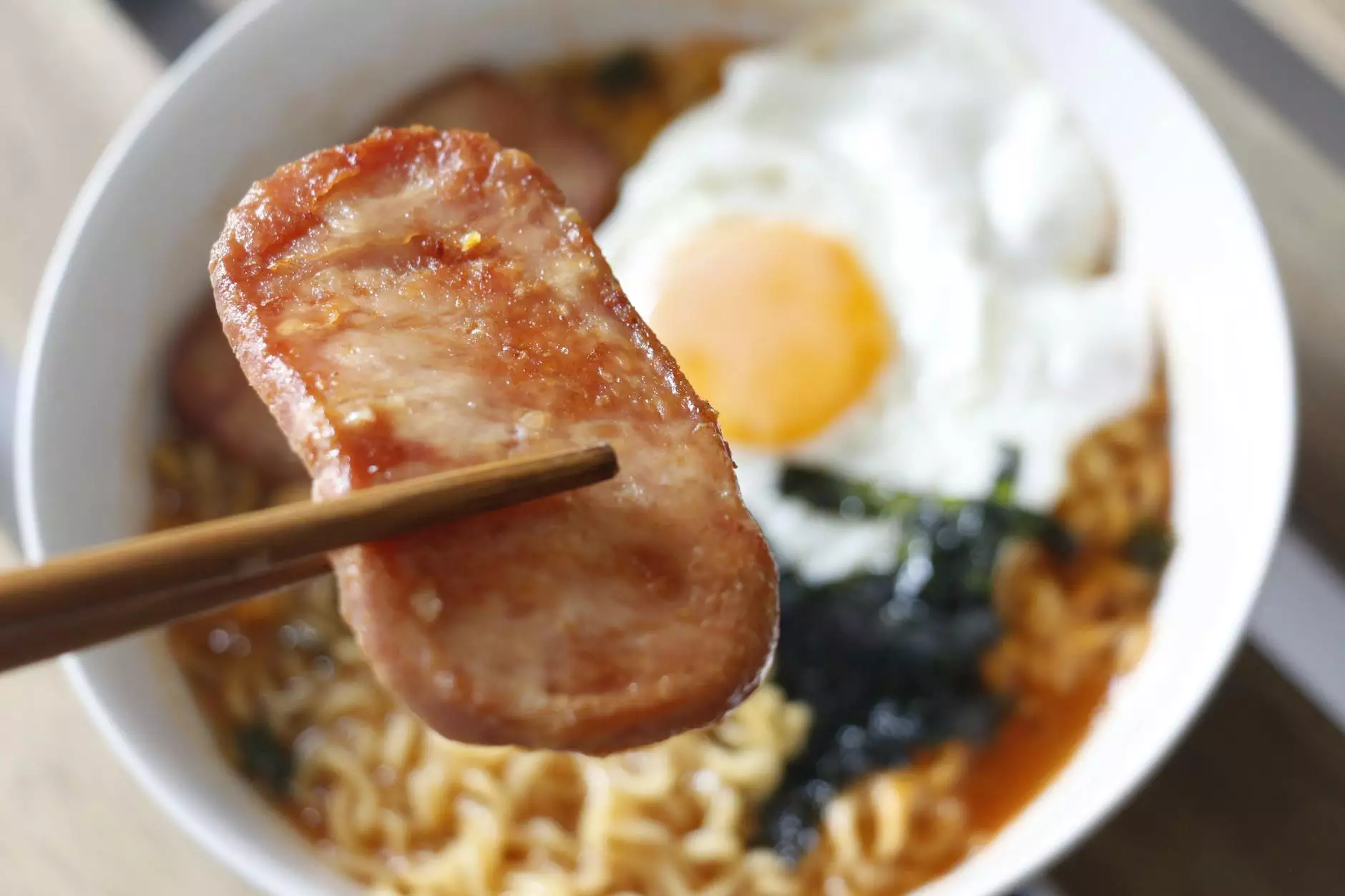
In today's digital age, managing email effectively is crucial for both individuals and businesses. The massive influx of emails every day can pose significant challenges, particularly when it comes to spam. This is where spam filtering machine learning comes into play, offering innovative solutions to an age-old problem. In this comprehensive guide, we will delve into how machine learning technology revolutionizes spam filtering, enhancing both IT services and security systems.
Understanding Spam and Its Impact
Spam emails are unsolicited messages, often sent in bulk, with the intent to advertise products or services, spread malware, or phishing attempts. The sheer volume of spam can not only clutter inboxes but also pose serious security risks to businesses. Estimates suggest that up to 50% of all emails sent daily are spam. This statistic highlights the necessity for robust filtering systems to protect users and maintain productivity.
The Traditional Approach to Spam Filtering
Traditionally, spam filtering has relied on rule-based systems where certain keywords or phrases are flagged as spam. These systems, while somewhat effective, have significant limitations:
- Inflexibility: Static filters can be easily bypassed as spammers continuously adapt their tactics.
- False Positives: Legitimate emails can wrongly be flagged as spam, leading to important communications getting lost.
- Manual Updates: Filters need constant updates to keep up with new spam trends.
The Emergence of Machine Learning in Spam Filtering
Machine learning introduces a transformative approach to spam filtering by utilizing data and algorithms to detect patterns and anomalies. Unlike traditional methods, machine learning systems can learn from new data inputs, continuously improving their filtering accuracy.
How Machine Learning Works in Spam Filtering
The process of applying spam filtering machine learning involves several key steps:
- Data Collection: Large datasets of both spam and legitimate emails are gathered to train the machine learning model.
- Feature Extraction: Specific features, such as sender information, subject lines, and email content, are analyzed to identify distinguishing characteristics of spam.
- Model Training: Using algorithms like Naive Bayes, Decision Trees, and Neural Networks, the model learns the differences between spam and legitimate emails.
- Testing and Validation: The model is tested with new data to assess its accuracy and refine its predictions.
- Deployment: Once validated, the model is deployed in real-time email filtering applications.
Key Advantages of Machine Learning for Spam Filtering
Spam filtering machine learning offers numerous advantages over traditional methods:
1. Enhanced Detection Rates
Machine learning models can achieve higher detection rates due to their ability to understand complex patterns within data. This makes it more challenging for spammers to bypass filters, as the algorithms learn from each new spam tactic.
2. Continuous Improvement
Once deployed, machine learning models improve over time, learning from new data inputs and user behavior to refine their filtering processes.
3. Reduced False Positives
With advanced pattern recognition, machine learning systems significantly reduce the chances of legitimate emails being flagged as spam, ensuring that important communications reach their intended recipients.
4. Adaptability
Machine learning algorithms can quickly adapt to new spam trends, reducing the need for manual updates and allowing for more responsive email management systems.
Implementing Machine Learning for Effective Email Management
Businesses looking to integrate spam filtering machine learning into their IT services can follow several steps:
1. Choose the Right Solution
Various platforms and tools provide machine learning capabilities for spam filtering. Options such as Google’s TensorFlow, Microsoft Azure ML, and open-source libraries like Scikit-learn can be considered based on business requirements.
2. Train Your Model
Ensure a comprehensive dataset is available for model training. The quality and diversity of the training data will significantly impact the model's effectiveness.
3. Monitor Performance
After implementation, it is essential to monitor the model's performance continuously. This includes tracking detection rates, false positives, and user feedback to optimize the model.
4. Implement User Training
Educating users about the spam filtering system helps them understand the rationale behind flagged emails and reduces the likelihood of them ignoring important alerts.
Practical Applications of Machine Learning in Spam Filtering
The application of spam filtering machine learning extends beyond email management. Here are some practical use cases where this technology can make a significant impact:
1. Corporate Email Security
Corporations rely heavily on email for communication. Implementing machine learning spam filters helps protect sensitive information and ensures compliance with regulatory standards.
2. E-commerce Platforms
Online stores can minimize fraudulent email communications and enhance customer trust by effectively filtering out spam from legitimate customer inquiries and transactions.
3. Financial Institutions
Banks and financial entities benefit from advanced spam filtering technologies to protect clients from phishing scams, thereby safeguarding their privacy and financial information.
The Future of Spam Filtering with Machine Learning
The future of spam filtering machine learning holds enormous potential. As machine learning technology advances, we can expect:
- Integration with AI: Further integration of artificial intelligence will enable even smarter spam filtering solutions.
- Multi-Layered Security: Combining spam filtering with other security measures like firewalls will create a comprehensive defense against cyber threats.
- User Personalization: Systems that use user behavior and preferences to tweak filtering rules will offer more tailored solutions to individual needs.
Conclusion
In conclusion, spam filtering machine learning is not just a revolutionary technology; it is a necessity for managing the overwhelming volume of emails faced by businesses today. By implementing advanced machine learning techniques in spam filtering processes, organizations can enhance their security systems, improve productivity, and ensure that legitimate communications are prioritized.
As we move forward into a more digitized world, the effective management of emails through intelligent spam filtering will remain a critical component of IT services, providing businesses with the tools they need to thrive in an increasingly competitive landscape. Embrace the power of machine learning to transform your email management strategy and protect your business against the evolving threat of spam.