Understanding the Importance of Data Labeling Tool in Software Development
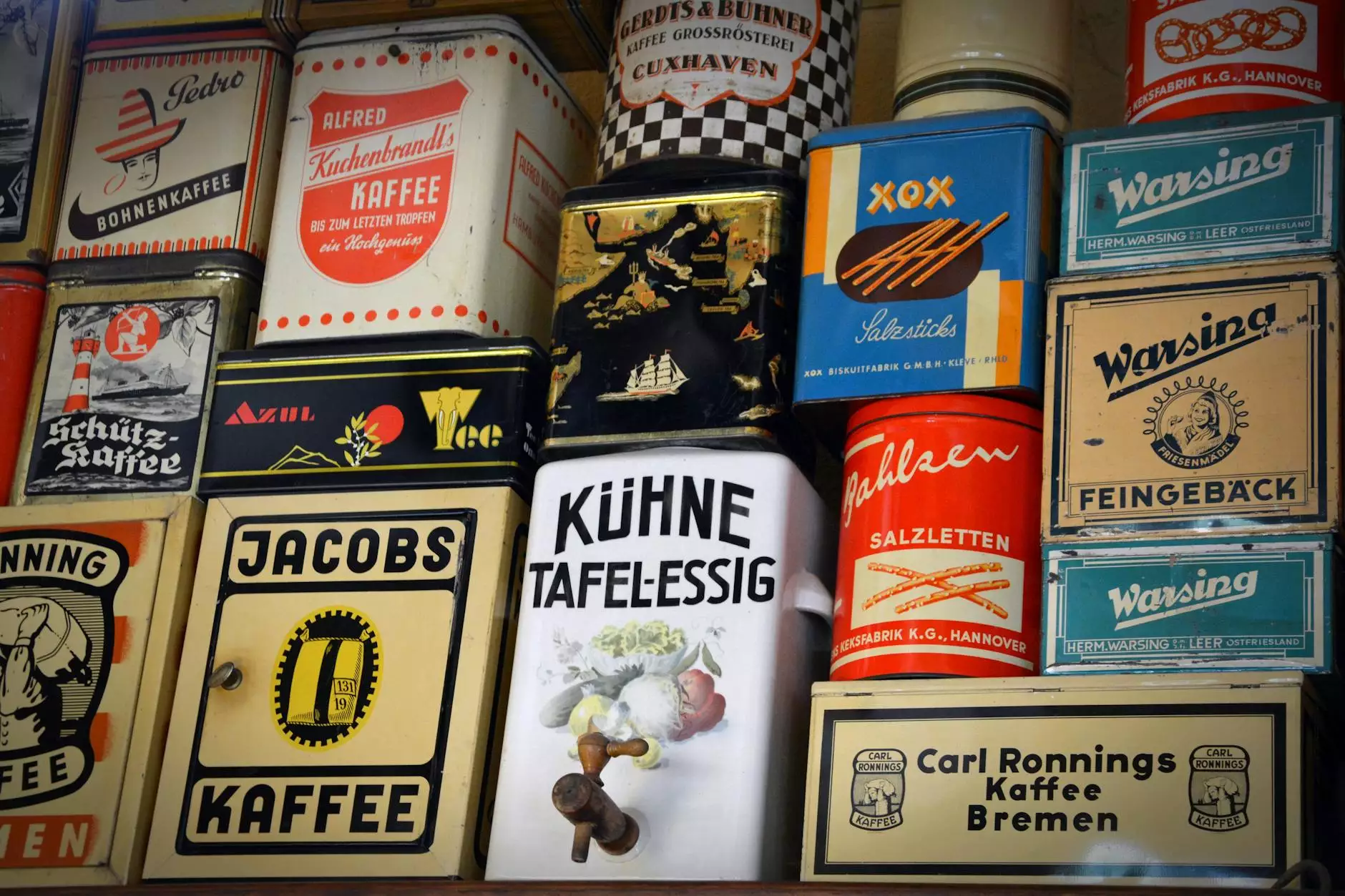
In the modern digital arena, data is not just an asset; it's the cornerstone of successful applications and services. As businesses continue to harness the power of artificial intelligence (AI) and machine learning (ML), the demand for effective data preparation becomes paramount. At the heart of this preparation lies a critical component: the data labeling tool.
What is a Data Labeling Tool?
A data labeling tool is software that enables the process of annotating data to create training sets that are essential for training machine learning models. This annotation can involve various tasks such as image tagging, text classification, or audio transcriptions. By applying labels to raw data, these tools help machines learn and make predictions based on the information they are fed.
Why is Data Labeling Crucial for AI and ML?
Data labeling serves as the bridge between raw data and actionable insights, playing a pivotal role in the development of AI and ML models. Here's why it's crucial:
- Quality of Data: High-quality, labeled data significantly enhances the performance of machine learning algorithms.
- Accuracy: The performance of AI models largely depends on the accuracy of the training data labels. Incorrectly labeled data can lead to biased results.
- Efficiency: Automated data labeling tools improve efficiency during data preparation, allowing developers to focus more on model building.
- Scalability: With the exponential growth of data, scalable solutions for data labeling are essential to maintain project timelines.
The Types of Data Labeling Tools
Understanding the types of data labeling tools available can help businesses select the right option that fits their needs:
1. Manual Labeling Tools
These involve human annotators who manually label the data. While they can achieve high accuracy, they are often time-consuming and costly.
2. Automated Labeling Tools
With advancements in technology, automated tools are now available that can label data quickly and efficiently, but they may require human oversight.
3. Semi-Automated Tools
These tools allow for a combination of machine-based labeling and human verification, providing a balanced approach to data annotation.
4. Domain-Specific Tools
These tools are tailored for particular types of data (image, video, text, audio) and cater to specific industries, enhancing accuracy and relevancy.
Benefits of Using Quality Data Labeling Tools
Investing in quality data labeling tools can yield numerous benefits for software developers and businesses, such as:
- Reduced Time to Market: Streamlined data preparation accelerates the development cycle, allowing for faster deployment of AI solutions.
- Improved Model Performance: Correctly labeled training data leads to better accuracy and performance of AI models, ultimately improving end-user satisfaction.
- Cost-Effectiveness: Efficient labeling processes lower labor costs and reduce the financial burden associated with data preparation.
- Enhanced Collaboration: Many labeling tools offer collaborative features that allow teams to work together seamlessly, improving communication and workflow.
Best Practices for Data Labeling
While implementing data labeling tools, it's essential to follow certain best practices:
1. Define Clear Guidelines
Establishing clear labeling guidelines ensures consistency and reduces ambiguity among annotators, leading to more accurate results.
2. Utilize Quality Assurance
Incorporating quality assurance processes, such as regular audits of labeled data, helps maintain the integrity of your training datasets.
3. Foster Annotations Feedback
Encouraging annotators to provide feedback during the labeling process can uncover potential pitfalls and improve overall accuracy.
4. Invest in Continuous Learning
As labeling technologies evolve, stay informed of updates and innovations to ensure your practices remain effective and efficient.
Keymakr: Leading the Way in Data Labeling Solutions
At keymakr.com, we understand that quality data is the backbone of successful AI and ML applications. Our data labeling tools are designed to streamline the labeling processes, making it easier for businesses to extract valuable insights from their data.
Our Features Include:
- User-Friendly Interface: Our intuitive platform allows users to navigate easily, reducing onboarding time and increasing productivity.
- Customizable Workflows: Tailor workflows to meet your specific project requirements, ensuring a smooth labeling process.
- Multi-Format Support: Whether working with images, text, or audio, our tools support a wide array of data formats.
- Advanced Collaboration Tools: Facilitate real-time collaboration among team members, ensuring everyone is on the same page.
- Robust Analytics: Get insights into your labeling process with our analytics tools, helping you identify bottlenecks and optimize workflows.
The Future of Data Labeling
As technology continues to advance, the future of data labeling tools looks promising. Key trends to watch include:
1. Increased Automation
Machine learning algorithms are becoming more sophisticated, allowing for more tasks to be automated in the data labeling process.
2. The Rise of Crowdsourcing
Crowdsourced labeling solutions are gaining traction, offering an efficient way to manage large datasets by leveraging a global workforce.
3. Expansion of AI-Based Tools
AI-driven tools are expected to improve labeling accuracy and speed, making it essential for businesses to keep an eye on emerging technologies.
4. Enhanced Integration with Other Tools
Future data labeling tools are likely to incorporate advanced integration capabilities with other data processing and analysis tools, creating a seamless workflow.
Conclusion
The significance of data labeling tools in software development cannot be overstated. As businesses increasingly rely on data-driven insights, having a robust labeling process becomes essential. By utilizing tools like those provided by keymakr.com, organizations can ensure they remain competitive, innovative, and capable of harnessing the true potential of their data.
In a world where data is the new oil, investing in the right data labeling tool and adopting best practices will not only enhance your machine learning initiatives but also bolster your company's capability to innovate and thrive in an ever-evolving market.